Suppose your decision problem is to determine when to do the bulk of your
driving in order to minimise your chances of dying in a car
crash. If you looked at the data for road fatalities in both the
US and Europe you will find something rather curious. The fewest
fatalities occur in the winter months (January and February)
while the most occur in the height of summer. In other words
there are fewest fatalities when the weather is at its worst and
when presumably the roads are at their most dangerous. If you
apply traditional statistical regression techniques using this
available data you will end up with a simple predictive model
like THIS:
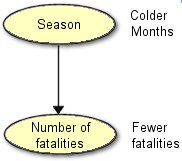
Colder months yield fewer fatalities. From a risk perspective this model would
provide irrational decision making since it would suggest that if you want
to minimise your probability of dying in a car crash you should do your
driving when the roads are at their most dangerous. The problem is that
this model provides no explanatory power at all.
What
we know is that there are a number of causal factors that do much to explain
the apparently strange statistical observations, so what we need is a causal
model like the one below. Clearly the season influences the weather, which
in turn influences road conditions. When the road conditions are bad people
tend to drive slower, so road conditions influence the speed at which people
drive. The danger level is at its highest when people are driving fast
and the road conditions are bad. Both the season and the weather influence
the number of journeys made - people generally make more journeys in summer
and will generally drive less when weather conditions are bad.
The
actual number of fatalities is influenced not just by the danger level
but also by the number of journeys. If relatively few people are driving,
albeit dangerously, there will be relatively few fatalities.
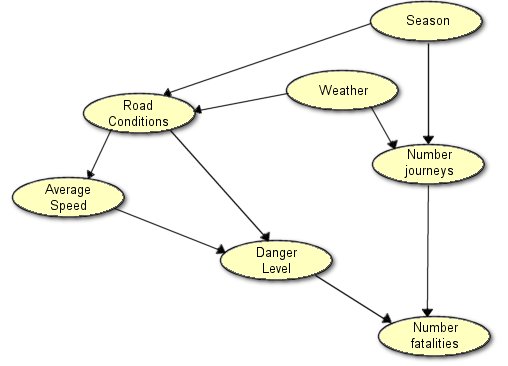
Using
this kind of model, which happens to be an example of a Bayesian Network
(BN), we can fully explain the statistical observations and also use it
to make sensible decisions about risk. For example, the only factors that
we can control ourselves here are the speed we drive and the number of
journeys we make. It turns out that if we keep both of these fixed then
the probability of a fatality in winter is greater than that in
summer – a rational observation, but one that was beyond the naïve
regression model. In fact, you can use the BN model to provide proper risk
assessment and reduction decision support – it will tell you that you can
minimise the probability of a fatality by driving slowly and driving when
the weather is good.
|